Member-only story
Deploying dbt Projects at Scale on Google Cloud
Containerising and running dbt projects with Artifact Registry, Cloud Composer, GitHub Actions and dbt-airflow
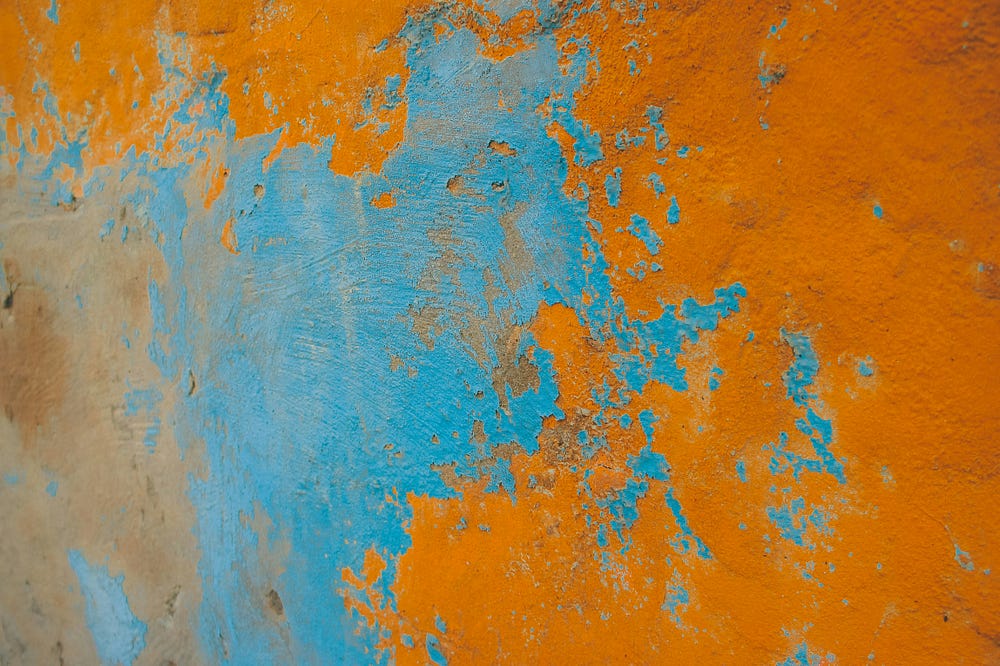
Managing data models at scale is a common challenge for data teams using dbt (data build tool). Initially, teams often start with simple models that are easy to manage and deploy. However, as the volume of data grows and business needs evolve, the complexity of these models increases.
This progression often leads to a monolithic repository where all dependencies are intertwined, making it difficult for different teams to collaborate efficiently. To address this, data teams may find it beneficial to distribute their data models across multiple dbt projects. This approach not only promotes better organisation and modularity but also enhances the scalability and maintainability of the entire data infrastructure.
One significant complexity introduced by handling multiple dbt projects is the way they are executed and deployed. Managing library dependencies becomes a critical concern, especially when different projects require different versions of dbt. While dbt Cloud offers a robust solution for scheduling and executing multi-repo dbt projects, it comes with significant investments that not every organisation can afford or find reasonable. A common alternative is to run dbt projects using Cloud Composer, Google Cloud’s managed Apache Airflow service.
Cloud Composer provides a managed environment with a substantial set of pre-defined dependencies. However, based on my experience, this setup poses a significant challenge. Installing any Python library without encountering unresolved dependencies is often difficult. When working with dbt-core
, I found that installing a specific version of dbt within the Cloud Composer environment was nearly impossible due to conflicting version dependencies. This experience highlighted the difficulty of running any dbt version on Cloud Composer directly.
Containerisation offers an effective solution. Instead of installing libraries within the Cloud Composer environment, you can containerise your dbt projects using Docker images and run them on Kubernetes via Cloud Composer. This approach keeps your Cloud Composer environment clean…